Profile
![]() |
Victor Czech, M.Sc. |
Publications
Retargeting Visual Data with Deformation Fields
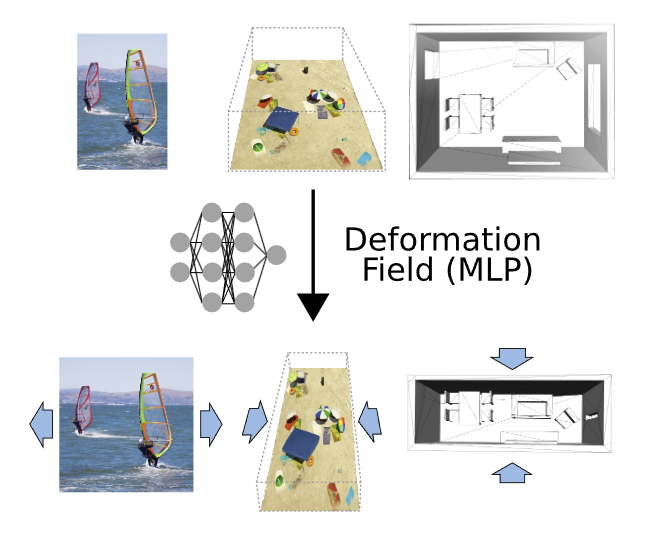
Seam carving is an image editing method that enables content- aware resizing, including operations like removing objects. However, the seam-finding strategy based on dynamic programming or graph-cut lim- its its applications to broader visual data formats and degrees of freedom for editing. Our observation is that describing the editing and retargeting of images more generally by a deformation field yields a generalisation of content-aware deformations. We propose to learn a deformation with a neural network that keeps the output plausible while trying to deform it only in places with low information content. This technique applies to different kinds of visual data, including images, 3D scenes given as neu- ral radiance fields, or even polygon meshes. Experiments conducted on different visual data show that our method achieves better content-aware retargeting compared to previous methods.
Intuitive Shape Editing in Latent Space
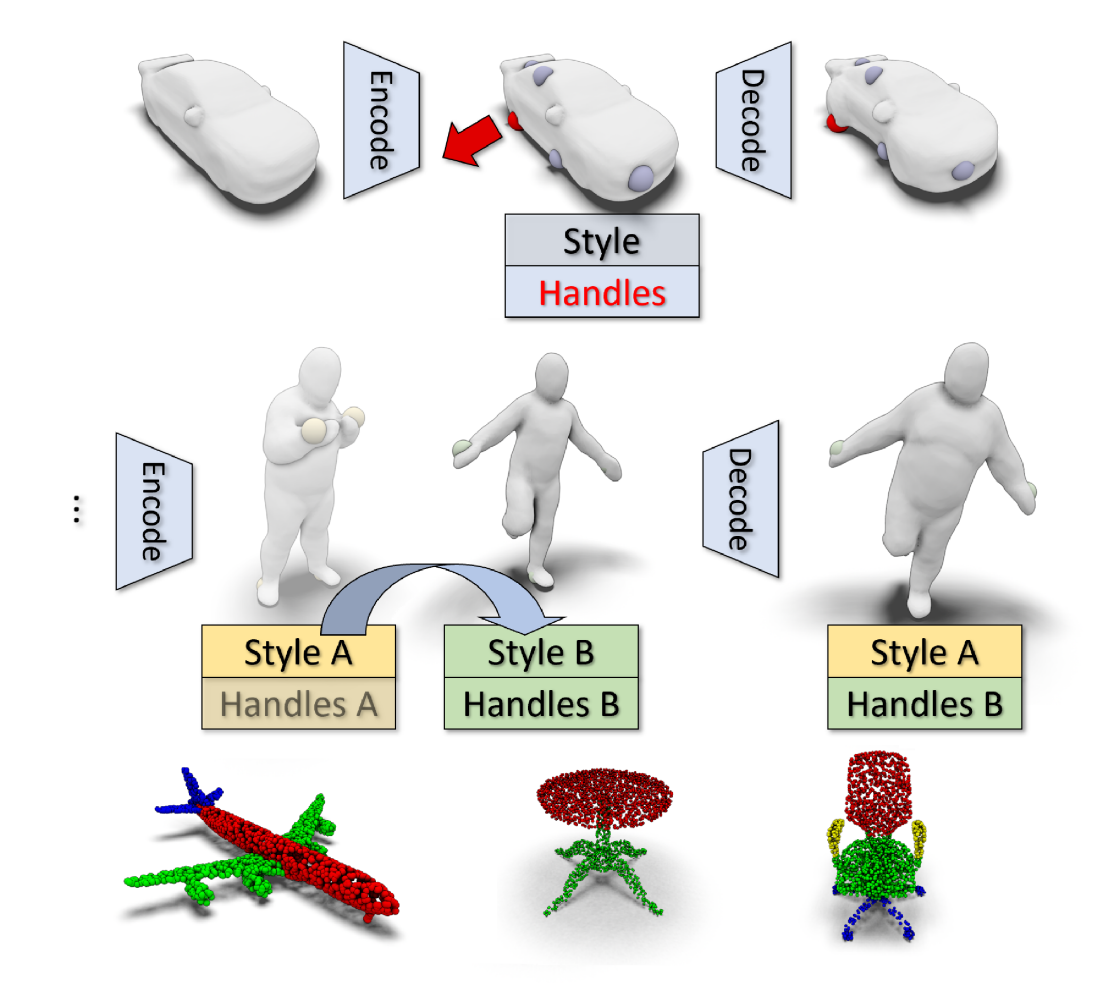
The use of autoencoders for shape editing or generation through latent space manipulation suffers from unpredictable changes in the output shape. Our autoencoder-based method enables intuitive shape editing in latent space by disentangling latent sub-spaces into style variables and control points on the surface that can be manipulated independently. The key idea is adding a Lipschitz-type constraint to the loss function, i.e. bounding the change of the output shape proportionally to the change in latent space, leading to interpretable latent space representations. The control points on the surface that are part of the latent code of an object can then be freely moved, allowing for intuitive shape editing directly in latent space. We evaluate our method by comparing to state-of-the-art data-driven shape editing methods. We further demonstrate the expressiveness of our learned latent space by leveraging it for unsupervised part segmentation.