Profile
|
Gregor Kobsik, M.Sc. |
Working on various topics at the intersection of Geometric Modeling, Shape Analysis and Machine Learning with a focus on Deep Shape Representation for Shape Analysis, Modeling, and Reconstruction. My goal is to utilize unsupervised or self-supervised deep learning methods to gain more insight into the composition and nature of geometric objects.
Currently, I am researching deep neural networks for Geometry Abstraction. Furthermore, I am supervising multiple thesis researching methods for 3D Shape Representation and Generation, Partial Symmetry Detection as well as 3D Structure and Relationship Detection.
Thesis Supervision:
- Geometry Abstraction using Pre-Trained Image Segmentation Models (Bachelor ongoing)
- Evaluation of Partial and Approximate Symmetry Detection for 3D Geometry (Bachelor 2024)
- Regressing the Attention Matrix to Learn Similarity Relationships in 3D Models (Bachelor 2024)
- Efficient Octree Shape Generation with RWKV: A Linear Scaling Transformer Alternative (Bachelor 2023)
- An Unsupervised Deep Neural Network for 3D Shape Cuboid Abstraction, Segmentation and Partial Symmetry Detection (Bachelor 2023)
- Learning 3D Shape Generation with Octree Value Quantized Deep Implicit Functions Transformer (Bachelor 2023)
- Clustering 3D Models with DeepDPM (Bachelor 2022)
Teaching:
- Data Analysis and Visualization (WS 2024)
- Seminar: Various Topics in 3D Deep Learning (SS 2024)
- Data Analysis and Visualization (WS 2023)
- Shape Analysis and 3D Deep Learning (SS 2023)
- Seminar: Various Topics in 3D Deep Learning (WS 2022)
- Datenstrukturen und Algorithmen (SS 2022)
Publications
Octree Transformer: Autoregressive 3D Shape Generation on Hierarchically Structured Sequences
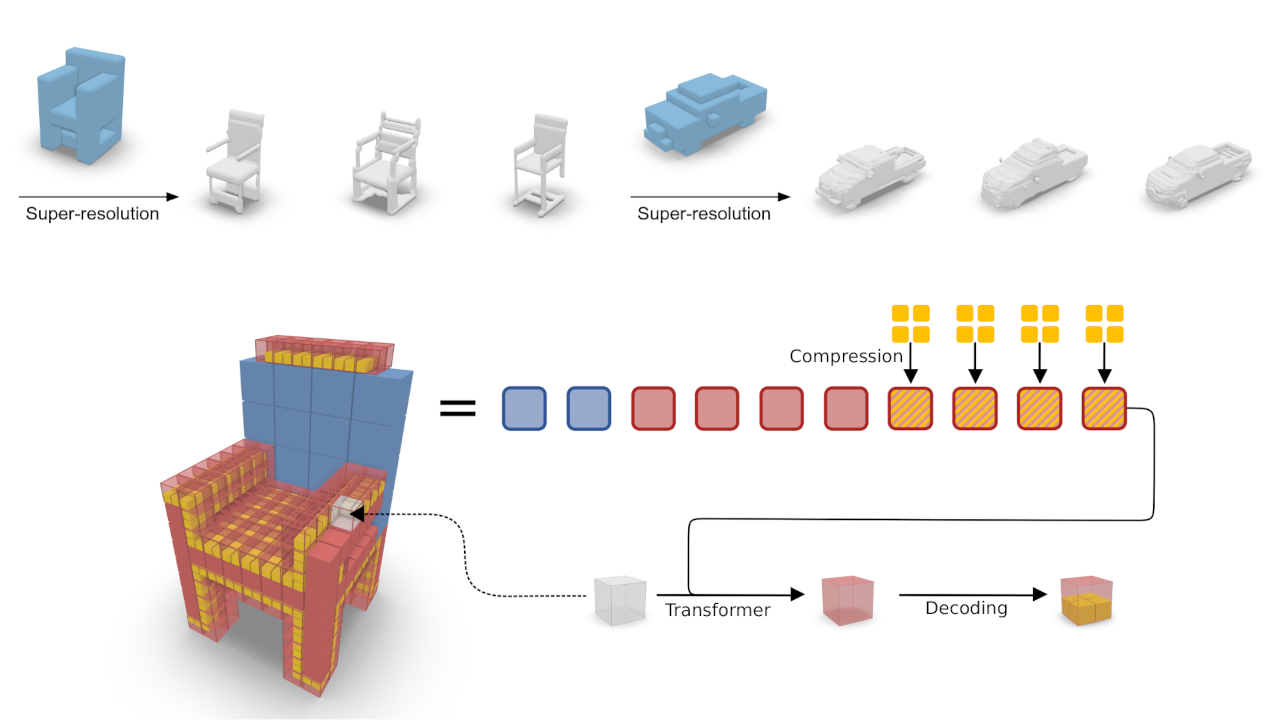
Autoregressive models have proven to be very powerful in NLP text generation tasks and lately have gained pop ularity for image generation as well. However, they have seen limited use for the synthesis of 3D shapes so far. This is mainly due to the lack of a straightforward way to linearize 3D data as well as to scaling problems with the length of the resulting sequences when describing complex shapes. In this work we address both of these problems. We use octrees as a compact hierarchical shape representation that can be sequentialized by traversal ordering. Moreover, we introduce an adaptive compression scheme, that significantly reduces sequence lengths and thus enables their effective generation with a transformer, while still allowing fully autoregressive sampling and parallel training. We demonstrate the performance of our model by performing superresolution and comparing against the state-of-the-art in shape generation.
@inproceedings{ibing_octree,
author = {Moritz Ibing and
Gregor Kobsik and
Leif Kobbelt},
title = {Octree Transformer: Autoregressive 3D Shape Generation on Hierarchically Structured Sequences},
booktitle = {{IEEE/CVF} Conference on Computer Vision and Pattern Recognition Workshops,
{CVPR} Workshops 2023},
publisher = {{IEEE}},
year = {2023},
}